Big Data-based Equipment Flaw Detection System
Technical framework
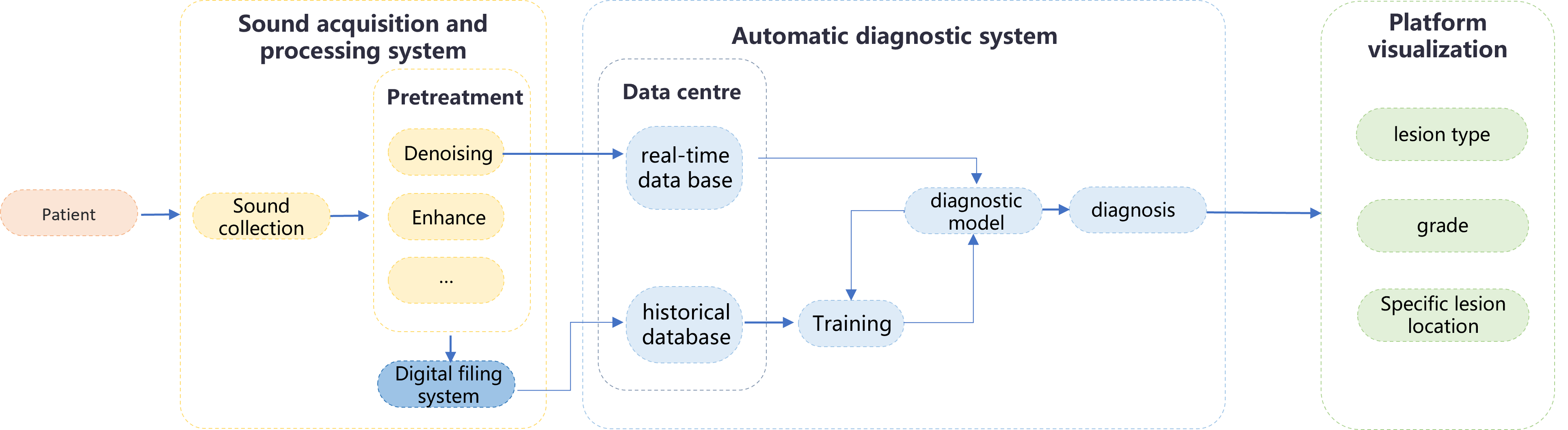
Application Scenarios
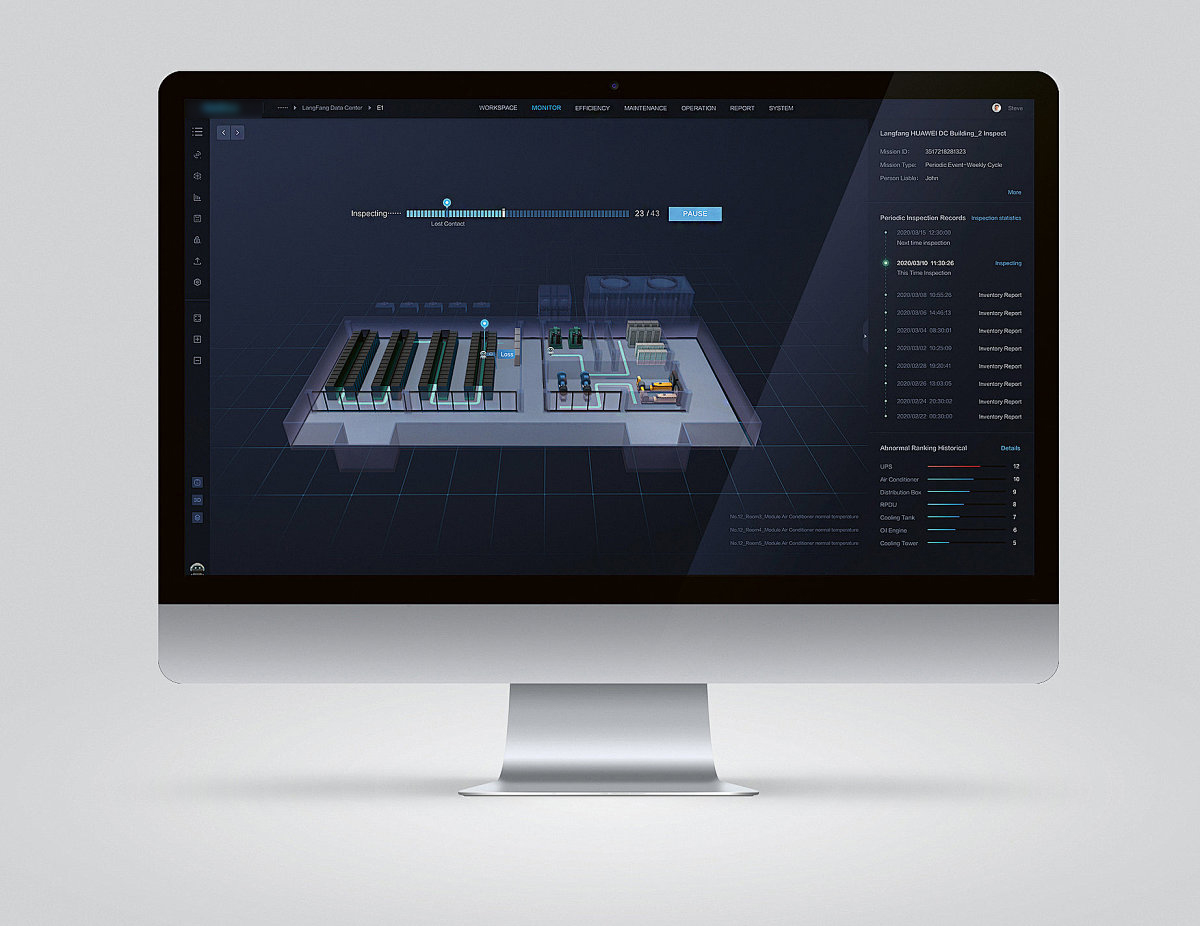
Description
- Our Big data-based equipment flaw detection system is designed to automatically detect flaws in equipment by collecting and analysing operating data such as operating time, temperature, and sound. Maintenance records are integrated with the operating data to generate training data, and machine learning is used to analyse the relationship between the data and equipment failures.
Application Scenarios
- Currently, most equipment flaw detection is done manually, leading to low quality and efficiency and safety hazards for operators. This system aims to address these issues using artificial intelligence algorithms to extract fault characteristics, determine causes, and generate fault resolution suggestions, helping operators quickly identify fault points and reduce workload. The system is divided into two parts: a passive fault diagnosis system, which uses fault signals and codes from the equipment's control system for real-time diagnosis, and an active fault diagnosis system, which uses high-precision sensors to actively analyse control signals, vibration signals, and temperature sensor signals for active maintenance. It is commonly used in the flaw detection of large equipment such as locomotives, and the comprehensive data it uses allows for more accurate diagnosis results.